Six Sigma, a methodology that has revolutionized quality management across industries, traces its roots back to the pioneering efforts of Motorola. In the 1980s, Motorola embarked on a mission to enhance customer satisfaction by eliminating defects and delivering products on time. The company initially focused on manufacturing defects but soon realized the importance of addressing latent defects hidden within product designs.
In the realm of Six Sigma, the Greek letter sigma symbolizes the standard deviation of a process. For context, a 3 sigma process variation results in 66,800 defects per million opportunities, while a 6 sigma process variation leads to a mere 3.4 defects per million opportunities. This stark contrast highlights the impact of process variation on defect rates.
It is involved not only applying statistical tools but also fostering a culture of continuous improvement and data-driven decision-making across all levels of the organization.
Similar to martial arts, Six Sigma requires extensive training and offers different levels of expertise – Green Belts, Black Belts, and Master Black Belts. Black Belts, in particular, play a crucial role as they possess both coaching skills and quantitative analysis proficiency.
Early Adopters: General Electric's Success Story
GE's commitment to Six Sigma involved a holistic approach, incorporating statistics and a disciplined methodology known as DMAIC: Define, Measure, Analyze, Improve, and Control.
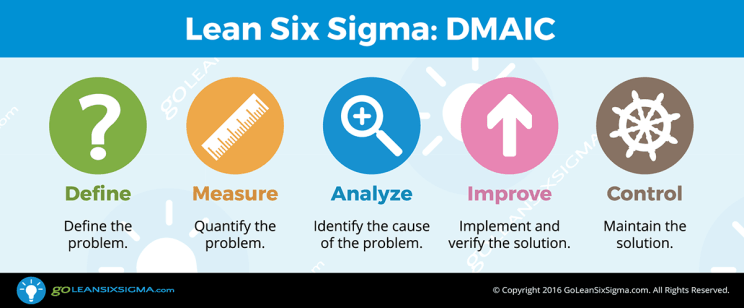
From defining customer-driven objectives to structured improvement cycles, process capability and control, process design, and organized training, Six Sigma embodies a comprehensive and disciplined methodology aimed at delivering sustained excellence in product quality and operational efficiency.
By integrating IoT devices into processes, organizations can collect real-time data, enabling more precise monitoring and analysis. This could lead to proactive defect prevention, predictive maintenance, and further optimization of processes.
Emerging technologies like advanced analytics and machine learning hold promise for the future of Six Sigma. These technologies can enhance predictive modeling, allowing organizations to identify potential issues before they occur.
Commitment to quality and innovation in Agriculture
GreenHarvest (a fictional AgroCompany) embraced Six Sigma to redefine its processes and enhance the quality of its plant nutrition products. The DMAIC cycles allowed GreenHarvest to identify areas for improvement systematically. For instance, by measuring and analyzing the nutrient composition of its products, the company could fine-tune formulations to meet customer specifications more precisely.
By designing robust processes with narrow tolerances, the company ensured that its products consistently met stringent quality standards. Process capability studies became instrumental in predicting and preventing deviations, contributing to a significant reduction in defects. AI-powered analytics provided insights into crop performance, allowing the company to fine-tune its products based on real-time data.
Before launching a new product, GreenHarvest conducted extensive field trials, analogous to the DMAIC cycles, ensuring that only the highest-quality solutions reached farmers.
Challenges in Implementing Six Sigma
- Resistance to Change: Employees and management may be accustomed to existing processes and may resist alterations.
- Data Availability and Accuracy: Investing in training programs and creating a culture of continuous learning can mitigate this challenge.
- Training and Skill Gaps: Implementing Six Sigma requires a workforce equipped with the necessary skills. Investing in training programs and creating a culture of continuous learning can mitigate this challenge.